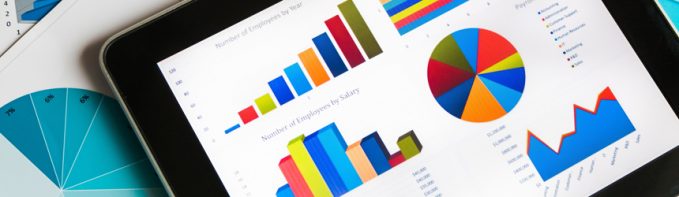
By Cassandra Balentine
Major technology trends transition the way businesses function, including big data and the cloud. The manner in which data is stored is an important business consideration. Data lakes are one method of storing data, typically in its native format.
A modern data lake enables organizations to efficiently store, manage, access, and generate value out of data stored in both on premise storage infrastructures as well as in the cloud, points out Tajinder Pal Singh Ahluwalia, product marketing lead, unstructured data storage, Dell. He says modern data lakes enable data to be consolidated in the right manner, allowing organizations to apply next-generation analytics and AI technologies to generate value from this data.
Lake in the Clouds
Technology trends, including the cloud and big data have created enormous amounts of data, which is commonly stored in data lakes. New technology trends, including the internet of things (IoT), artificial intelligence (AI), and live streaming data, there is now more data in the data lake and the desire to analyze it in new and different ways.
Jerome Sandrini, SVP, head of big data and cyber security, Atos North America, believes the cloud has the same effect on data lakes than pretty much anything else—more simplicity, flexibility, and consumption-based model. “This clearly helps accelerating the growth while IoT and AI/machine learning (ML) generate always more data, driving the need for more robust scalable across enterprise data lakes infrastructures,” he says.
Data lakes are evolving to handle different modes of data movement—streaming for example—and different formats of data that are either consumed or generated by the IoT and AI projects, points out Sriperumbudur.
He adds that enterprises are embracing cloud applications—including CRM and ERP systems—for cost and speed advantages, therefore moving tons of big data to the cloud. Modern data lakes in the cloud benefit from the same advantages—they are faster, cheaper, and more agile.
The rise of IoT, AI, and other computing advancements have businesses looking closer at data lakes for two main reasons—the massive volume of data that connected applications and devices generate, and the abundance of innovative opportunities to use that data. “As the data volume grows and the data itself becomes more important, people will develop new ways to inject it into applications to make it useful,” comments Ilya Pupko, chief architect, Jitterbit. This will lead to an evolution in the way companies collect and share data with internal and external consumers that we see with a shift towards virtual data lakes.”
“Though managing a data lake or data hub to drive business insights used to be expensive, moving data lakes to the cloud have eased some of those pains along with elastic infrastructure,” explains Vamshi Sriperumbudur, head of marketing, big data and analytics portfolio, Informatica. Cloud-based data lakes tend to offer better availability than what is guaranteed on premises, faster time to value, and time to deploy for new projects, data sources, and applications already cloud-based and scalable.
With the growth of the cloud, more companies look for cloud-based or hybrid data lake architecture. “Many organizations are now leveraging object store technologies like Amazon S3 and Azure Blob Store,” says Mark Shainman, marketing director, Teradata.
While AI and ML is a key use case once you build your modern data lake, AI itself can be used to build an intelligent enterprise-ready data lake. “Ingest, integrate, catalog, prep, govern, secure, relate—these functions are critical for building a modern data lake and can be automated using AI,” says Sriperumbudur.
Ahluwalia adds that data analytics and AI initiatives are important vehicles for organizations to leverage to unlock the value of their data capital and gain new insight that can identify new opportunities to increase efficiency and expand business. To do this, he says data must be consolidated into a modern data lake that eliminates data silos and allows data to be added and accessed from a wide range of sources and applications, using a variety of protocols.
In terms of the IoT, Sriperumbudur says high-volume data at high velocity is the nature of data produced by the IoT. If you don’t process streaming data immediately, it will go stale very quickly. Modern data lakes must balance the collection as well as the processing of data at the edge and centrally, leveraging a sense-reason-act framework for streaming data.
“The IoT is an important driver of unstructured data growth and a rich source of data capital for many organizations,” shares Ahluwalia.
These trends all entail data and processes that are well suited for a data lake architecture. They’ve pushed the evolution of data lakes by growing together, alongside the data lake market. Because more organizations realize that data lakes can help with these modern data trends, they consider data lakes a critical tool for today’s analytical environments, says Dale Kim, senior director, products/solutions, Arcadia Data. “For example, data lake architectures share many of the same advantages of the cloud. Interest in cloud capabilities have steered organizations toward data lake architectures. The scalability of data lakes makes them ideal as the foundation for AI/ML processing, especially considering the amount of data necessary to create effective models. Data lakes also handle the types of data that IoT implementations create well—massive volumes of fast, streaming data from numerous sources. Data lakes are built to handle these modern data types at scale.”
Benefits
Data lakes are associated with many benefits, here we discuss them in contrast with another popular storage method—data warehouses.
Ahluwalia says traditional data warehouses are typically limited to operational, structured data managed using a few specific applications accessed by only a few people. “A modern data lake consolidates unstructured data, eliminates only costly storage silos across the organization, and supports a range of applications and workloads, including data analytics with a single storage infrastructure. With a data lake, organizations can increase efficiency, simplify management, and unlock the value of their data assets by putting the right data in the hands of the business users who can derive value from it.”
Shainman says the benefit that some people feel the data lake has over the traditional warehouse is that the data lake does not have the rules, structure, and governance of the traditional warehouse. “This allows users to dump and store huge amounts of data cheaply and quickly in the lake without dealing with the rules and governance and sometimes cost that the data warehouse requires. Unlike the traditional warehouse, the data in the lake can have limited structure and schema. What defines and differentiates data lake and data warehouse is architecture and function, not technology,” he says.
A data lake is a function and architecture decision. When it does come to architecture and technologies that are traditionally used, such as relational databases, object stores, or Hadoop, they are complimentary architectures and technologies. “Initial exploration can be done in the lake to see if the data even has value and warrants deeper analysis, and then can be moved to a high performing platform with structure and governance of a warehouse or analytics platform,” says Shainman.
Kumar Thangamuthu, senior technical architect, SAS, points out that data moves through a data lake much faster than a data warehouse, reducing latency and providing faster time to analyzed data. Additionally, data lakes use schema-on-read approach, accelerating insights and lowering the cost of acquiring new types of high-volume data, sensor data, social media data, and clickstream data.
Data lakes are flat by nature, and users don’t need to think upfront about the type of queries or service the data will be leveraged for, because we are not stuck with one specific data model or view of the data. “In the same way, data lakes can store similarly structured and unstructured data—indexing, data engineering and management, and setup of specific views or queries can be built later based on the specific business case,” offers Sandrini.
Data lakes help you manage any type of data, unstructured data as well as structured and semi-structured data. Examples of the former include data from IoT, mobile applications (apps), social media, and click stream, while the latter data is from databases and business applications. “Data lakes are meant for a variety of use personas; data scientists, data analysts, data engineers, and data stewards, in addition to business analytics. With data lakes you can analyze data in real time and generate machine learning models to provide new business insights not possible with data warehouses. Data lakes enable self-service—like data democratization, as they are IT enabled instead of IT managed,” says Sriperumbudur.
“Although there are significant differences between data lakes and data warehouses, they both complement warehouse and other downstream applications. Meanwhile, data warehouse serves as a repository of integrated, historical data that is dimensionally modelled and can be queries in an ad hoc manner by concurrent business users,” shares Thangamuthu.
Kim says overall, data lakes help reduce IT involvement and the associated costs that data warehouses require. “Popular objectives today around self-service and data consumerization are realized with analytics deployments on data lakes.”
Limitations
While the benefits discussed above are experienced with data lakes, there are limitations to consider as well.
Data lakes lack strong governance, metadata, security, and other standard features of enterprise software, though managed data lakes are starting to address some of these limitations. “As companies move beyond piloting advanced analytics projects to run data lakes in production and at scale, they have found the software tools quite expansive; they also require investments in people and products required to manage the open source functionality and infrastructure,” offers Thangamuthu.
Sandrini points out that data lakes do not address the real value customers are looking for. “They are a set of tools useful to store the data centrally and keeping all flexibility to leverage this data later on for business purposes. But, without a clear and good data strategy and understanding of the data sources, with the right teams in terms of data engineering and data science, all this data can become completely useless, irrelevant, and create more issues than added value. The cost for an enterprise data lake can also be quite high for the enterprise with many hidden costs depending on the volume, type of data tiers used, tools, and number and type of sources for ingestion.”
For structured—and in some cases, semi-structured data—data warehouses can be faster in producing business reports. If not all catalogued, enriched, related, secured, and governed—data lakes become data swamps, offers Sriperumbudur.
Data warehouses are valuable for the most responsive, production analytic dashboards and this is where they excel over data lakes. If you have critical data that leads to immediately actionable insights in BI-style analytics, a data warehouse will give you the highest performance and interactively that you need. “As long as you use your data lake for use cases that aren’t the sweet spot for data warehouses, you will likely get great value. So if your use case is more about broad ranges of data types and sources, and interactively within a few seconds is satisfactory, the data lake will be a great choice,” offers Kim.
Kim adds that data lakes are a much newer technology than data warehouses, so a potential limitation for them is around the availability of technical expertise. Because modern data lake technology is only just making its way into mainstream consideration, there will necessarily be a smaller talent pool available for organizations that wish to implement a data lake. But as the number of incriminations in the market continue to grow, the talent pool will naturally grow with it.
From a technology perspective, traditional data lake technologies and engines can be limited in concurrency and performance. From an architectural standpoint, Shainman says the lake can be lacking when it comes to rules, structure, and governance.
It is important to remember that a modern data lake requires a storage infrastructure that can store data from a wide range of sources while supporting a broad range of applications and workloads. “To enable this, a storage platform used to support a data lake needs to provide multiprotocol support,” shares Ahluwalia.
Modern data lakes must also incorporate both on premise data as well as data stored in the cloud. “To support rapid growth, a data lake must be able to scale easily and efficiently without disruption. A data lake supported by a scale out storage infrastructure would address this important need,” says Ahluwalia.
Pupko believes the limitations associated with data lakes and warehouses should be thought of on a spectrum. “On one side you have data warehouses, where data is shipped from various placed, cleaned, and it’s turned into a well-defined, well-structured set of data. On the other side, standard data lakes are quite murky. Raw data is essentially dumped there without being cleaned up first, and to get what you need you’ve got to do a fair amount of work to fish for it. The need to move and store the data, and the effort to fish for that data are quite expensive limitations—if not blockers—or many businesses. Virtual data lakes overcome the cleanup issues that limit the usefulness of data lakes by organizing and formatting data as it comes in, and they avoid the limits associated with actually moving the data to another location that come with the data warehouse concept. Virtual data lakes do have their own limitations in that they can be though to set up, but because they are virtual systems, businesses can start small and scale them easily as the project grows.”
Verticals
Modern data lakes can be set up to be both vertical and horizontal, meaning industry vertical specific or line of business specific or both.
Sriperumbudur offers the example of a trusted healthcare data lake aggregates data from hundreds of hospitals in a major health system to deliver timely, trusted data as needed, and reduce risks associated with regulatory reporting.
Another would be a supply chain use case at a leading life sciences manufacturer uses a healthcare data lake leveraging self-service data preparation to find, access, and prepare data for analytics, says Sriperumbudur.
The differences are mainly on the data sources and the type of data including the update frequency—streaming versus batch. It is simple to understand that healthcare and financial services will be targeting very different data sources with requirements very much linked to the specifications of the industry, shares Sandrini. “In the industry, IoT/OT products will typically require very specific edge gateways for data ingestion and preprocessing.”
The basic nature of the data lake concept is industry agnostic. “A data lake is a strong system that can store large amounts of data in its original format until required by advanced analytic and visualization applications to derive insights,” says Thangamuthu. “Verticals simply differ by how they use the data—in other words, how the data is transformed to be used by a specific organization or industry.”
Kim says solutions will differ by data types, dashboard types, and outputs—but the overall architecture is consistent across industries. This makes data lakes so powerful as they can often address multiple, distinct use cases in a single deployment. “In general, data lake architectures include distributing data across multiple machines in a cluster to handle fast, incoming data from a variety of sources. They are often built to handle information sharing and enabling data agility.”
Differences also pertain to regulations around different types of data. “Typically, organizations will look to use the same architecture best practices regardless of the industry in which they operate. If you think about the vastly different types of use cases—cybersecurity or marketing analytics, for example—they will largely have the same architectural foundation even if the end value and insights those organizations desire are different.”
“For certain industries, such as finance, bad collections of data have the potential to be major security disasters, but it’s an issue that really spans across all verticals,” shares Pupko. For instance, think of consumer data privacy, GDPR, the California Privacy Act, and other sets of regulations threaten real consequences if users’ data is stored inappropriately or shared with the wrong people. “Virtual data lakes need to be structured in a way so that you secure the data and can properly limit access to certain sensitive data and offer different permissions depending on the requestors.”
Lake Life
Data lakes evolve with modern technology trends, offering both pros and cons for organizations looking for a cost-effective and efficient method to store and manage ever-increasing data.
Jan2019, Software Magazine