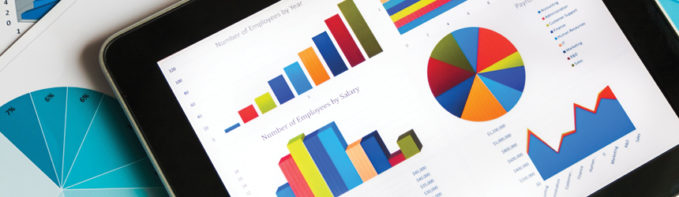
By Cassandra Balentine
Acompany’s data is only useful when clean and well maintained. Although the importance of data is increasing for businesses of many sizes and functions, managing it is an ongoing challenge for all.
Core Functions
To help address data issues, data quality software tools are available to verify, complete, and examine data to enhance its value and potential. Data quality software tools help provide accurate and complete data for their organizations. Typical functions include profile, transform, cleanse, identify, match, merge, enrich, and import. Overall, data quality tools enable businesses to understand, standardize, and monitor data over the course of its lifecycle.
These tools typically exist to provide the ability to examine data and determine its quality. “This assessment may be statistically based, business-rules based, heuristic based, or use some other method,” says Tyler Warden, VP of solution management, BackOffice Associates. “In the end, a data quality tool is all about looking at data and determining its quality level and telling the users which data is good and which is bad.”
With data quality tools, organizations profile and interact with dashboards that enable users to visualize data. “As the problem of bad data has become more pervasive within organizations, people outside of the data analyst role need to be able to access and understand just how bad data records are. Because of this, dashboards have become extremely important. They provide line of business (LOB) users with the ability to actually profile data and see the inaccuracies as well as help the betterment of that data and see how it’s making an improvement. It’s no longer good enough to just see how bad your data is, but rather you need to be able to address those issues head on,” states Thomas Brence, director, product marketing, Informatica.
Data quality tools also provide the ability to verify, correct, and enrich contact data in real time, including U.S. and global address, name, phone, and email info, comments Greg Brown, VP of marketing, Melissa Data. “Many enrichments can be added—demographic, firmographic, psychographic, and geographic—to blend big data streams for added value and insight,” he adds.
Ciaran Dynes, VP of product marketing, Talend, notes that data quality tools provide simple connectivity to a range of data sources as well as easy to use functionality for data quality assessment and visualization, parsing, standardization, and cleaning—including location-based standardization, matching and relationship identification, metadata management, and administrative controls for managing data quality processes. “Good solutions should also enable ongoing quality monitoring, the ability to easily integrate data from a range of external sources to improve data completeness, and a workflow and user interface to allow business users to perform their own data quality related tasks as well as fulfill stewardship requirements.”
Ultimately, enterprise information management solutions enable a successful digital business. Companies can deliver trusted, complete, and relevant data that everyone can use and support the critical capabilities to architect, integrate, improve, manage, associate, and archive all information. “Existing technologies lack the capabilities that allow organizations to easily gain access to and govern big data and to make use of cloud platforms for information storage and cost reduction. These tools allow companies to mash up data form social networks with their enterprise information sources; transform and combine data to reduce the time and complexity of preparing data for analysis; understand what data exists within the enterprise and the relationship of this data with data in other systems; and help users quickly analyze and monitor data quality,” says Philip On, VP marketing for enterprise information management, SAP.
Driving Demand
As businesses rely more on data every day, the need for good, clean data is more important than ever. It is also critical that these functions are available in real time.
Primarily, the sheer volume of data creates the need for tools that support its management, security, and value.
Matthew Magne, global product marketing manager for big data management, SAS, says that as emerging big data technologies like Apache Hadoop mature and find a place in the data landscape, issues like data quality and data security have grown in importance. “Risks to brand reputation caused by data breaches and data privacy issues as well as regulatory and compliance mandates have also driven the need for data governance and data quality. Once policies are defined, most data governance implementations begin with an assessment of data assets, their level of data quality, and monitoring of data and processes to ensure it’s accurate. Finally, analytics have seen greater adoption in organizations in terms of driving better business decisions. Accurate and consistent data is essential to analytics processes to drive accurate decisions.”
On suggests that while companies move toward the Internet of Things to help modernize their workplace and embark on a digital transformation, there is often a price that comes with it as well—an influx of data and complexity. Having access to more information is always beneficial. “However, if companies want to share this information and use it in real time to help capture new business opportunities, they must equip themselves with tools that help manage and improve data quality. Only then are companies able to weed through the influx of data, extract the best value from it, and have a clear view of what’s relevant for the business process or decision at that point in time.”
“We see a growing need for active data quality processes—where the data is verified in real time, at point of entry, as opposed to rules-based data quality regiments,” comments Brown.
Automation is another factor driving the demand for better data, and consequently, data quality tools. Warden says data stewards want technology that automates and streamline a process that can be manual or homegrown.
Good Data for Good Business
Leveraging data for business insight is now essential to success. “It doesn’t matter the size of the business or the industry, everyone wants to better leverage their data assets,” says Erin Haselkorn, public relations manager, Experian Data Quality. “Businesses use data to find new customers, better understand customer needs, and increase retention. Those core business goals stretch across every department within an organization.”
Warden also sees an increasing understanding at top levels of the enterprise regarding the importance of high-quality data in executing high-quality business processes.
Christopher M. O’Brien, EVP, communication and shipping solutions, Neopost USA, agrees, pointing out that demand for data quality tools is often driven by someone in an organization having an “ah-ha” moment where the quality of data was the root cause of a particular business initiative or operation failing. “Poor data quality can result in an over-budget system migration project, ineffective reach, or a targeted marketing campaign, a poor customer experience due to erroneous information, inaccurate analytics leading to bad business decisions, and regulatory incompliance penalties in some industries.”
He adds that as companies mature, demand is driven by more proactive initiatives motivated by parts of the organization, such as a data governance team or CIO responsible for the defined data strategy in support of that organization’s goals. “The proactive measures may include Master Data Management, a point of entry data quality firewall, or data quality monitoring to support defined data standards and continuous improvement.”
“We’re seeing massive demand across the board for everything related to making the most of company data,” says Dynes. “This is clearly a reflection of the fact we are living in an increasingly data-driven world. Companies today win or lose based on how effectively they can leverage data. Across every industry, businesses are redefining markets, driving success largely based on data. Companies such as Uber, Netflix, General Electric (GE), and Amazon led the way and created a bit of a data arms race. Big bets are being made based on data, so that needs to be of the highest possible quality.”
Increased amounts of data and traditional data quality problems are only becoming more problematic. “If businesses do not carefully monitor the quality of their data, it can result in inconsistencies, duplication, and inaccuracies,” says Brence. “The issues that come from pulling data insight from bad data become more pervasive as the volume of data grows. In addition, new catalysts, such as regulations, have made organizations hyper-aware of bad data that had previously gone undetected. With the increase in data governance programs at organizations, these data quality issues can be more easily identified and addressed,” he adds.
Organizations that have felt the pain resulting from bad data are interested in preventing it in the future. “For example, if a shipment has to be recalled because the ‘should be refrigerated’ check box was not properly set, people ask questions and want to avoid these types of costly mistakes moving forward,” says Warden.
Haselkorn points out that the classic statement of “garbage in, garbage out” rings true when it comes to data. She says inaccurate information is damaging to the customer experience, operational efficiency, and ultimately, the bottom line. “Many organizations struggle with inaccurate data. In fact, our research shows that businesses believe that almost a quarter of their information is inaccurate. High percentage stems from the sheer volume of data, the number of people entering information, and the lack of a central data owner.”
Early Adopters
The necessity for good, clean data is not specific to one market or vertical, but some industries are more advanced in terms of their useful data lifecycle.
Haselkorn says data quality tools can benefit anyone. “Early adopters of data quality tools are typically those within an organization who are impacted the most by inaccurate data. For example, an email marketer maybe unable to reach subscribers because of a large number of inaccuracies, leaving them unable to perform their function. They may look for an email validation tool to solve that specific problem,” she recommends.
“When too much time or money is being spent on resolving data quality issues, the people asked with resolving those issues have been the first to look for a solution,” says Warden.
Early and/or prime adopters include commercial enterprises like Walmart, Bank of America, Northrup Grumman, GE, and many local, state, and federal government agencies.
Dynes says companies in retail and financial sectors were among the first adopters of data quality tools. “However, today we’re seeing broad support from mid to large enterprises across virtually all industries from healthcare and manufacturing to transportation.”
Brence points out that from a vertical perspective, the financial services industry has become a prime adopter of data quality tools. This is due to regulatory compliance and security requirements. Additionally, as healthcare regulations have advanced, the need for trusted data throughout the healthcare industry has advanced as well, putting an emphasis on tools that ensure high-quality data.
On says every organization needs real time access to data that can be trusted, and to have the flexibility to prepare for the unique needs of every department and user. “Companies are recognizing the need for a more streamlined system of processing data so they can get data that is complete, reliable, relevant, and usable,” he says.
“Early or prime adopters of these tools are in compliance-driven organizations like financial services or healthcare industries where there is a specific cost associated with not ensuring high levels of data quality for reporting,” agrees Magne. “Improved customer experience drives adoption in departments from retail stores to casinos,” he adds.
O’Brien brings the discussion to the mailing industry. “Print service providers have been leveraging data quality tools for decades. The demand was driven in part by regulations enforced by various postal authorities to reduce the amounts of undeliverable as addressed mail that was entered into the mainstream to help drive down the cost of mail delivery and in part by the industry’s desire to reduce operational cost associated with return mail and duplicate mail pieces.”
He explains that the mailing industry has been leveraging data quality tools for decades. “Most notably are tools to help ensure address quality via postal certified address correction software. The goal is a complete, correct, and standardized address for accurate, on time delivery as well as to meet requirements for postal discounts,” says O’Brien.
Shifting to the enterprise, O’Brien says early adopters of data quality tools were IT professionals. When operational and analytical data related issues are traced back to some system or database, resolution of those issues by default became the responsibility of the owner of those systems and databases. Data quality tools naturally came into play when the problems become too complex to be solved by a custom SQL script. “As organizations have become more technology and data dependent, IT project backlogs have swelled. At the same time, LOB professionals have become more data savvy. The result is more departmentally tailored datamarts with designated—formally or informally—departmental data stewards, that go-to person who really knows the data running a particular function within the organization.”
He says that these data stewards have become prime adopters for data quality tools to help ensure the success of goals and objectives that rely on accurate, complete, and up-to-date data. “The tools themselves have evolved to provide user friendly user experiences in support of this shift.”
Brence agrees, noting that data analysts are the biggest groups within organizations using data quality tools. He points out that as more organizations establish data governance programs and hire chief data officers, these new roles are also making use of these tools. “Employees with governance roles—from governance owners within individual business units—are all touching the data quality problem.”
For the Enterprise
When implementing data quality tools, IT should be at the helm. From there, depending on the scale needed, others in the organization can help manage the data lifecycle.
Dynes agrees, noting that central IT is the primary team for large scale data quality initiatives. However, more business teams execute smaller data quality projects independently, such as marketing scrubbing contact lists received from trade shows. “While I believe certain LOB teams can be successful with smaller-scale data quality projects, for the most part, IT needs to be the overall framework. This includes governance rules, defining roles and responsibilities, establishing the quality expectations of the organization, supporting best practices, and deploying the technical environment needed to support it all efficiently.”
Warden says that almost all data quality tools require some level of technical expertise for install and configuration, whereby a technical expert hooks the tool to the stores of data in the organization—database, flat files, data feeds, APIs, etc. “Once that connection is established, the work of defining what good data means to the enterprise begins. This step in the implementation process is best accomplished by business users, but some tools are more technically focused while others are more business focused.”
Haselkorn says data quality tools themselves need to be implemented across the organization, wherever data is collected, reviewed, and prepared for insight. However, she says that where tools are implemented is often not the biggest problem. “We look at data quality in terms of the people, processes, and technology that surround it. While a lot of organizations are implementing data quality technology, many are not getting it right and still having issues with inaccuracy. That is because all components of a data quality strategy are not in place and no one is taking responsibility for the overall quality of the organization’s data.”
Wherever data resides, there are going to be data quality issues. Brence says that no origination is perfect. “No one has a silver bullet and there isn’t a quick fix to bad quality data. You have to fix everything centrally all at once and that’s where we see roles like data steward come into play. However, this may be tangential to their current job responsibilities. For example, in healthcare, a data steward could be anyone from a member of the IT all the way to an end user—like a nurse—who actually can see data quality issues directly from the medical floors themselves. In this scenario, having a data steward that is also a nurse helps hospitals and healthcare organizations understand why and how bad data gets into the system. These stewards are typically the biggest proponents of the need for accurate data and the ones within the businesses that are caring for the quality of data—while also providing quality care to their patients.”
Brown sees data quality tools implemented at point of entry. He explains that enterprises typically have many entry points for data including websites, call centers, as well as customer relationship management. “Additionally, many enterprises will implement these tools as part of the process to ensure only clean, accurate, and updated data that enters the warehouse for distribution and use among the various business users,” he notes.
On feels data quality tools should be implemented at all levels of the enterprise. “This includes within the IT department, data stewards and scientists, and business users. Previously, the task for managing and improving data was placed with IT and they were left to figure out the problem with data quality. However, the truth is, because the business owns their data, they know what good data looks like to the business user. Whether someone works in marketing or sales, they understand their customer base better than anyone and are therefore in the better position to improve the data quality.”
Magne says data quality is strategically reactive as well as ad hoc. “For example, a marketing team realizes that their monthly mailing list needs cleansing. The updates, corrections, and removal of duplicates was never pushed back to the source systems. With increased computational power we’re seeing more of the processing being pushed down to database systems like Teradata or Hadoop. At the same time, real-time integration via Web services or REST API allows for integration and application of the same data quality rules within your most frequently used applications like customer relationship management or Salesforce Automation at the point of integration,” he adds.
Good, Clean Data
Data is increasingly essential to nearly every organization. In order to leverage the data properly, data quality tools are used to manage, authenticate, and enhance company data. These solutions continue to improve with the latest demands, offering real-time access, enrichment capabilities, and more. SW
Nov2016, Software Magazine